OUR THINKING
Our research and white papers provide concrete insights into our thinking and our technology
Neural Error Mitigation of Near-Term Quantum Simulations
Near-term quantum computers provide a promising platform for finding the ground states of quantum systems, which is an essential task in physics, chemistry and materials science. However, near-term approaches are constrained by the effects of noise, as well as the limited resources of near-term quantum hardware. We introduce neural error mitigation, which uses neural networks to improve estimates of ground states and ground-state observables obtained using near-term quantum simulations. To demonstrate our method’s broad applicability, we employ neural error mitigation to find the ground states of the H2 and LiH molecular Hamiltonians, as well as the lattice Schwinger model, prepared via the variational quantum eigensolver. Our results show that neural error mitigation improves numerical and experimental variational quantum eigensolver computations to yield low energy errors, high fidelities and accurate estimations of more complex observables such as order parameters and entanglement entropy without requiring additional quantum resources.
Finding Ground States of Spin Hamiltonians With Reinforcement Learning
Reinforcement learning (RL) has become a proven method for optimizing a procedure for which success has been defined, but the specific actions needed to achieve it have not. Using a method we call ‘controlled online optimization learning’ (COOL), we apply the so-called ‘black box’ method of RL to simulated annealing (SA), demonstrating that an RL agent based on proximal policy optimization can, through experience alone, arrive at a temperature schedule that surpasses the performance of standard heuristic temperature schedules for two classes of Hamiltonians. We investigate the performance of our RL-driven SA agent in generalizing to all Hamiltonians of a specific class. When trained on random Hamiltonians of nearest-neighbour spin glasses, the RL agent is able to control the SA process for other Hamiltonians, reaching the ground state with a higher probability than a simple linear annealing schedule. The success of the RL agent could have far-reaching impacts, from classical optimization, to quantum annealing and to the simulation of physical systems.
Access all our research and white papers
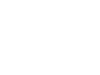
Research Papers
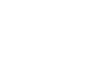