"As an industry leader in AI, 1QBit has developed XrAI, a 'co-pilot' clinicians can use to improve the accuracy and speed of diagnosing lung-related abnormalities."
Pneumonia, tuberculosis, and other respiratory infections remain the world’s most deadly communicable diseases and fourth leading cause of death.1 Chest radiographs, commonly called “X-rays” or “X-ray images”, are widely used to diagnose chest abnormalities. Interpreting a radiograph can be a challenge because every scan is unique—and interpretations can vary, even among experts. More than 3.5 billion radiographs are taken around the world each year.2 Artificial Intelligence has shown strong potential to assist radiologists in their challenging clinical workflows, one way being in helping them to interpret chest radiographs.
As an industry leader in AI, 1QBit has developed XrAI, a “co-pilot” clinicians can use to improve the accuracy and speed of diagnosing lung-related abnormalities. XrAI classifies and highlights abnormal regions in radiographs using deep learning (DL), a field within AI.
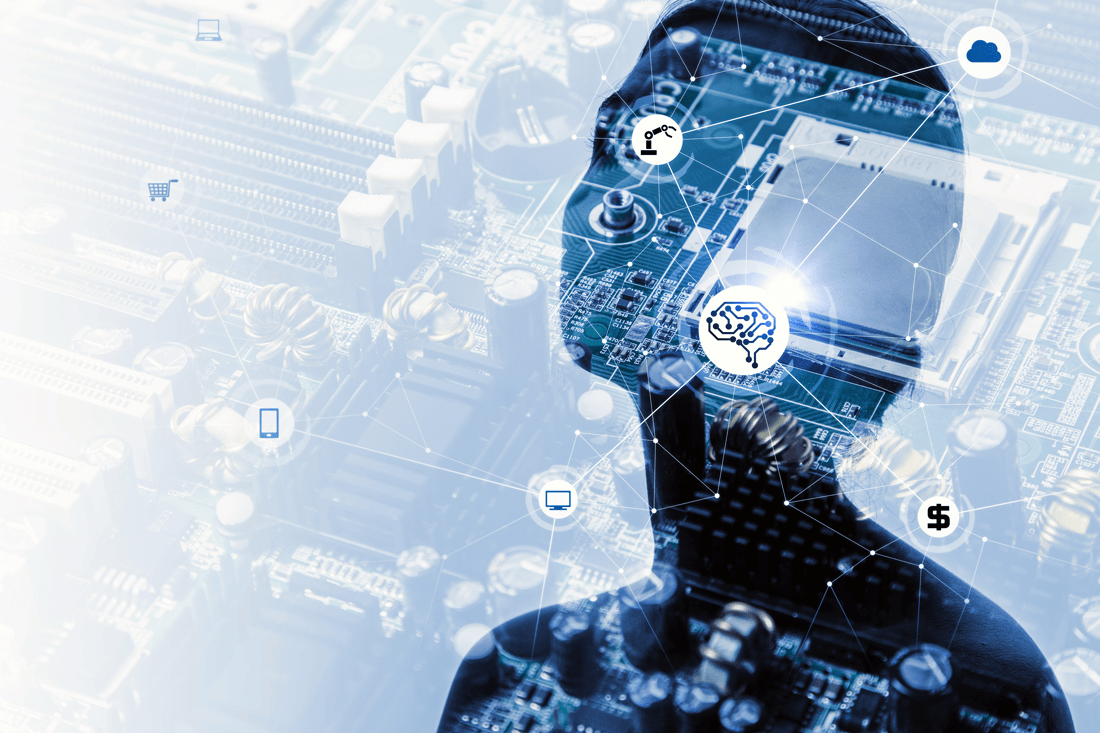
Four Steps Needed to Apply Deep Learning to Radiology
Deep learning involves designing computer models inspired by the networks in biological brains, models known as “artificial neural networks”. The goal is to train a DL model to make identifications much like a human might. Training the model with enough data will reinforce connections in the artificial “brains”, allowing accurate predictions to be made. One reason the development of DL models has received a lot of attention in recent years is their ability to be used for the detection of abnormalities in chest radiographs.
The First Step: Acquire enough data to train a DL model. There is a large amount of chest radiograph data available to the public. Among them, the largest dataset has 377,110 chest radiographs and 14 disease labels.3 The precisely defined disease classifications among the data, labels are used to train the DL model. Another dataset has 174 different disease labels and consists of more than 160,000 chest radiographs.4
The Second Step: Analyze the radiology reports corresponding to the radiographs to extract disease labels. One way to label the dataset is to assign radiologists to the task, but this requires significant time and resources. Labelling can be automated using an AI technique known as “natural language processing”, where a computer model analyzes data containing human language and is capable of extracting the contents of documents.
The Third Step: Train the DL model to predict new labels that will be used to find abnormalities in the radiographs. As the data consist of images, “convolutional” neural network models are used. These models are a class of DL networks that are effective at extracting features from images using computer vision models. “Heat maps”—representations of data using coloured graphics—can be generated that provide approximate locations of a given predicted abnormality, allowing for an improved diagnosis.
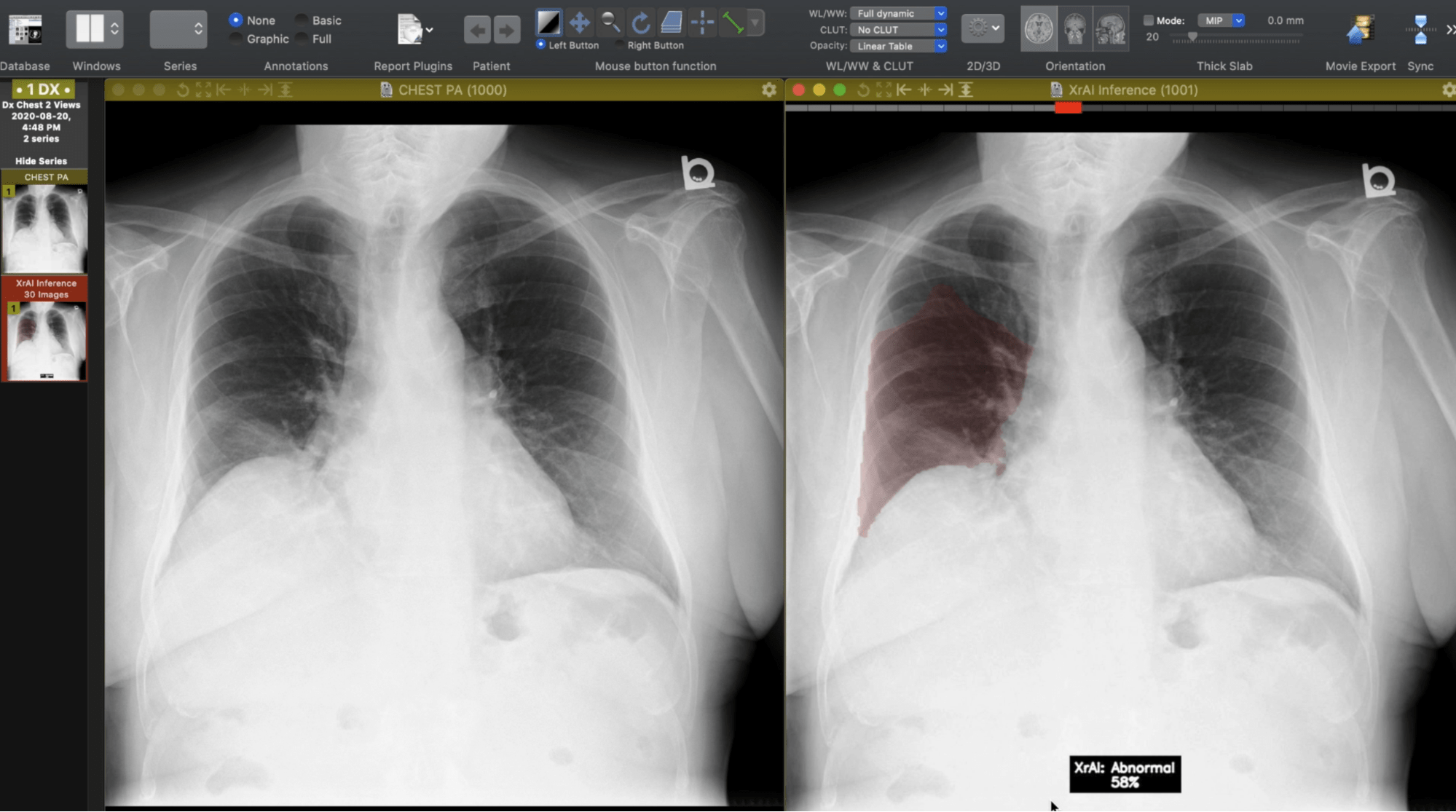
The Fourth Step: Deep learning models must be tested using performance metrics, such as accuracy, sensitivity, and specificity. The most common approach is to compare the performance of the model against the work of radiologists. To build accurate labels for the dataset being evaluated, most research suggests using experts’ manually annotated labels.5-7 The performance of DL models indicates promising results, as some chest abnormalities detection techniques have shown better performance than that of radiologists.5-7
Obstacles Encountered in Deep Learning Models
There are still some obstacles to overcome. For instance, massive amounts of labelled data are required for training DL models.
Another significant limitation is the requirement to validate a DL model for clinical implementation. Validation requires significant resources, including multi-institutional collaboration, on top of having large datasets. Obtaining these datasets can be difficult if companies wish to protect their intellectual property and keep the datasets proprietary.8
Future Opportunities in Deep Learning
Deep learning tools can assist clinicians in their practice, augmenting their ability to make accurate and faster diagnoses. Still, the critical role radiologists play in the diagnosis of diseases, along with the need for incidental observations, will not be diminished by these tools.
Learn more about XrAI, 1QBit’s co-pilot for clinicians, and stay up to date on 1QBit’s research and health care products by subscribing to our blog and following us on social media.
References
1 WHO, “The top 10 causes of death”, World Health Organization (2019).2 W. Paho, “World Radiography Day: Two-Thirds of the World’s Population has no Access to Diagnostic Imaging”, Pan American Health Organization (2012).
3 J. Alistair EW, T. Pollard, N. Greenbaum, et al., "MIMIC-CXR-JPG, a large publicly available database of labeled chest radiographs", arXiv:1901.07042 (2019).
4 A. Bustos, A. Pertusa, J. M. Salinas, et al., "Padchest: A large chest x-ray image dataset with multi-label annotated reports", Medical Image Analysis, 101797 (2020).
5 J. Irvin, P. Rajpurkar, M. Ko, et al., “CheXpert: A Large Chest Radiograph Dataset with Uncertainty Labels and Expert Comparison ”, In Proceedings of the AAAI Conference on Artificial Intelligence, 590–597 (2019).
6 A. Majkowska, S. Mittal, D. F. Steiner, et al., “Chest Radiograph Interpretation with Deep Learning Models: Assessment with Radiologist-adjudicated Reference Standards and Population-adjusted Evaluation”, Radiology, 421–431 (2020).
7 P. Putha, M. Tadepalli, B. Reddy, et al., “Can Artificial Intelligence Reliably Report Chest X-Rays?: Radiologist Validation of an Algorithm trained on 2.3 Million X-Rays”, arXiv:1807.07455 (2018).
8 M. P. McBee, O. A. Awan, A. T. Colucci, et al., “Deep Learning in Radiology”, Academic Radiology, 1472–1480 (2018).