With social media gaining popularity in the world of financial trading, there is interest in using the sentiment, or opinion, of many people automatically in algorithmic trading. For example:
“Market sentiment analysis can be used as triggers for trades that can reduce drawdown and increase return on investment.”
- How do announcements and social media coverage surrounding major events like the COVID-19 pandemic, an election, a UN resolution, or perhaps all of the above affect financial markets?
- How might you filter through all the chatter on Twitter or Reddit and quantify the overall sentiment of traders when analyzing market trends or making investment decisions?
Text sentiment analysis, or opinion mining, is a popular technique that uses artificial intelligence (AI) to try and answer questions like these.
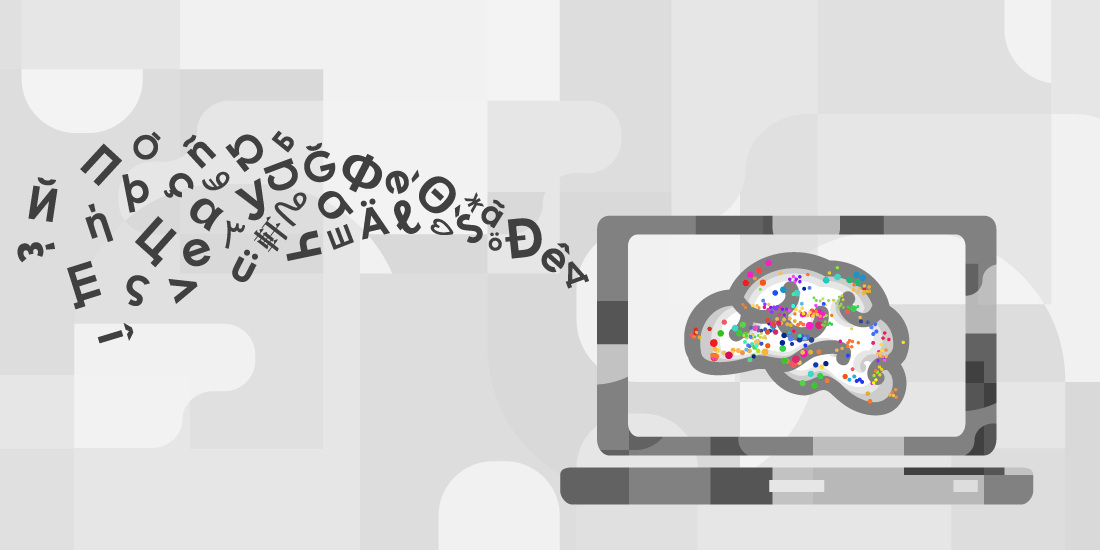
How does it work?
Text sentiment analysis uses a machine learning framework called natural language processing (NLP). In NLP, data is pre-processed to extract grammatical structure and to identify how words relate to each other with respect to syntax and semantics. Text is transformed into an array of numbers used by computer algorithms.
Machine learning algorithms are used for training a computer to ‘understand’ the interpretation of words. With enough data, statistical methods build ‘knowledge’ to discern the most meaningful features in the text data—like positive or negative sentiment.
How is sentiment analysis used in finance?
Constructing a reliable and consistent trading strategy is a keystone for investors. Automated trading strategies perform better with more robust input that indicates shifts or unusual activity within a market.
Text sentiment may have potential in estimating price movements. Input is taken from relevant social media and news feeds to calculate polarity scores, quantifying sentiment as positive, negative, or neutral.
Market sentiment is another type of sentiment analysis that quantifies the overall attitude of traders toward a particular market. It is calculated from actual market data, based on futures and options settlement data.
Both types of sentiment analysis were applied in a case study of the year 2020 regarding the US Treasury 10-Year Note futures (TYF). The year brought large fluctuations in the US economy due to the COVID-19 pandemic. The US Federal Reserve acted by adding more cash into circulation, more colloquially referred to as ‘quantitative easing, or ‘printing money’. Other major events include the UN Security Council Resolution 2532 regarding a global ceasefire and the US presidential election.
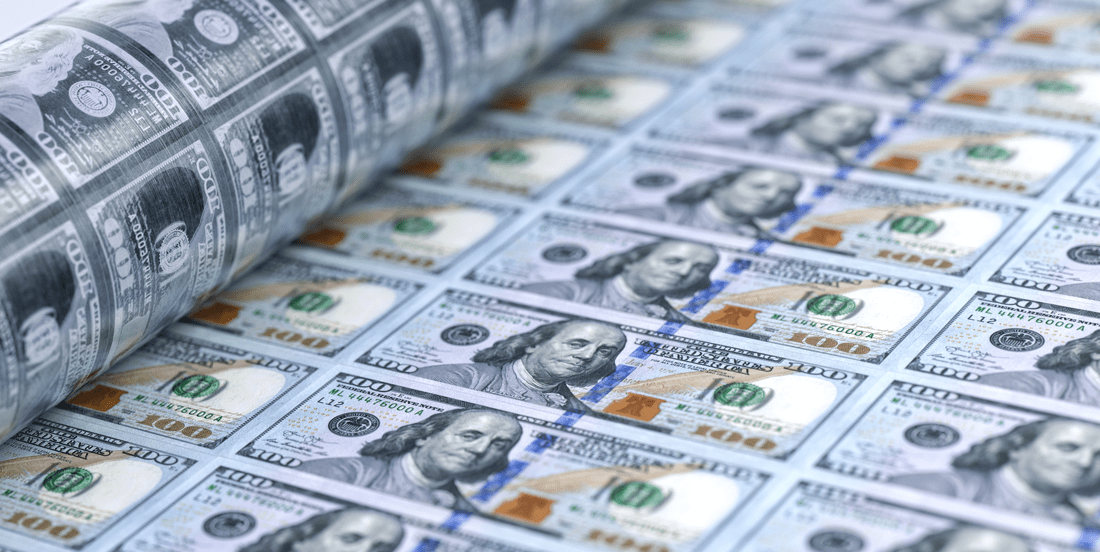
What did the sentiment analysis reveal about the economy?
For the text sentiment analysis, a model called Valence Aware Dictionary for Sentiment Reasoning (VADER) was used on raw speech data from the Federal Reserve.
It was found that the polarity score was a lagging indicator for changes in TYF, and would not necessarily provide better results than simply looking at changes in the cash in circulation. A more detailed analysis of this case study is available here.
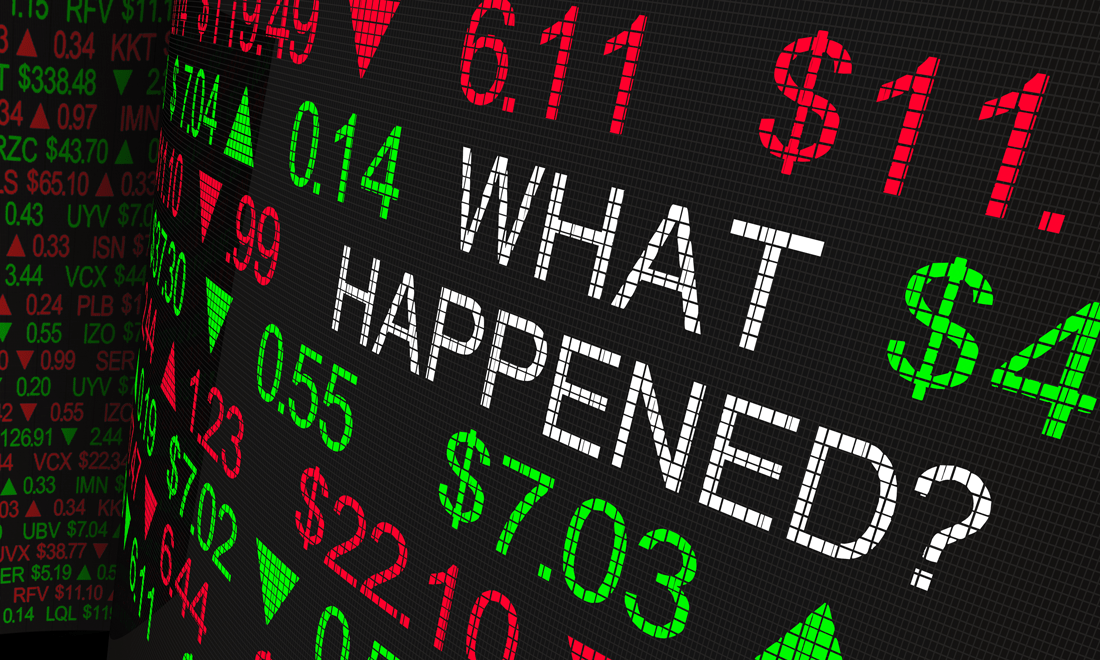
For the market sentiment, the CME Market Sentiment Meter (MSM) was used to calculate different market sentiment states across the same time period.
The MSM showed that the market was in an anxious state during the printing money period, resulting in a sharp spike in standard deviation, indicating a higher degree of risk for traders. The standard deviation quantifies risk: when it is larger, there is a higher chance of a larger price movement.
During the time of UN Resolution 2532, the MSM indicated the market was in a complacent state with a decrease in standard deviation. During the time of the US presidential election, the MSM indicated the market was in a conflicted state which preceded a rapid increase followed by a decrease in standard deviation.
In short, the MSM mixture distribution standard deviation shifted earlier than the polarity score found through the VADER analysis.
Although it is highly relevant in the age of social media, the study revealed that text sentiment analysis tends to be more reactionary to price changes, rather than being a source of price changes.
Note that Twitter sentiment has been applied in the algorithmic trading of cryptocurrency with some success.1 Using such open-source tools have a relatively lower barrier to entrance, but usually requires some skills and specific knowledge.2, 3
Market sentiment analysis showed more forecasting power and insight into shifts or unusual activity within a market. This information can be used as triggers for trades that can reduce drawdown and increase return on investment.
See here for more information on market sentiment and the MSM. If you are interested in making the MSM part of your trading strategy and would like to speak to an expert, contact us at msm@1qbit.com.
References
1 S. Colianni, S. Rosales, and M. Signorotti, “Algorithmic Trading of Cryptocurrency Based on Twitter Sentiment Analysis ”, Stanford University Research, (2015).2 T. A. Bohn, “Improving Long Term Stock Market Prediction with Text Analysis”, Western University Electronic Thesis and Dissertation Repository, (2017).
3 P. Lohman, “Portfolio investment strategy based on Twitter sentiment", Umeå University, (2017).