It is estimated that one billion radiologic examinations are performed every year worldwide,1 and this number continues to grow. Since every medical image is due the best analysis possible, radiograph interpretation is a daunting yet critical task requiring a significant amount of time, resources, and expertise. Among radiographs, chest X-rays (CXR) represent about 40% of all imaging procedures performed.2
"The vision of pAI is to be a low-cost AI radiology clinic to provide greater access to medical imaging for underserved communities that lack internet access and financial or medical resources."
XrAI is a deep learning3 model that can significantly help both radiologists and clinicians to identify lung abnormalities in CXR to improve the accuracy and timeliness of diagnoses. XrAI is a Class 3 medical device authorized by Health Canada and can be deployed on-prem, directly integrated into existing clinical information systems (e.g. Picture Archiving and Communications System—PACS), or as a service-based application over the cloud.
Researchers at 1QBit are extending the functionality of XrAI to see if the tool could be used to help diagnose lung conditions for people living in remote communities. Many of these communities do not have the infrastructure to support an on-prem PACS platform and have unreliable access—or even lack access—to the Internet.
The researchers developed this concept in a project that they named pAI (pronounced “pie”). The vision of pAI is to be a low-cost AI radiology clinic to provide greater access to medical imaging for underserved communities that lack internet access and financial or medical resources.
The idea is that a clinician could take a picture of a CXR with a smartphone camera and send it over a local network to a low-power, inexpensive edge device for processing using the XrAI model, and then the AI inference is returned to the smartphone.
The 1QBit team created a prototype of the pAI project at the (Society for Imaging Informatics in Medicine) SIIM Hackathon, where the team earned second place for their demonstration. 1QBit is the only industry partner from Canada that participated in the contest.
To run the prototype model, it was necessary to hack out parts of the full XrAI functionality that would be too heavy to run on the low-power edge device. The device chosen was a Raspberry Pi 4 Computer Model B (8GB). Raspberry Pi is a series of small single-board computers developed originally for teaching basic computer science in schools and in developing countries.
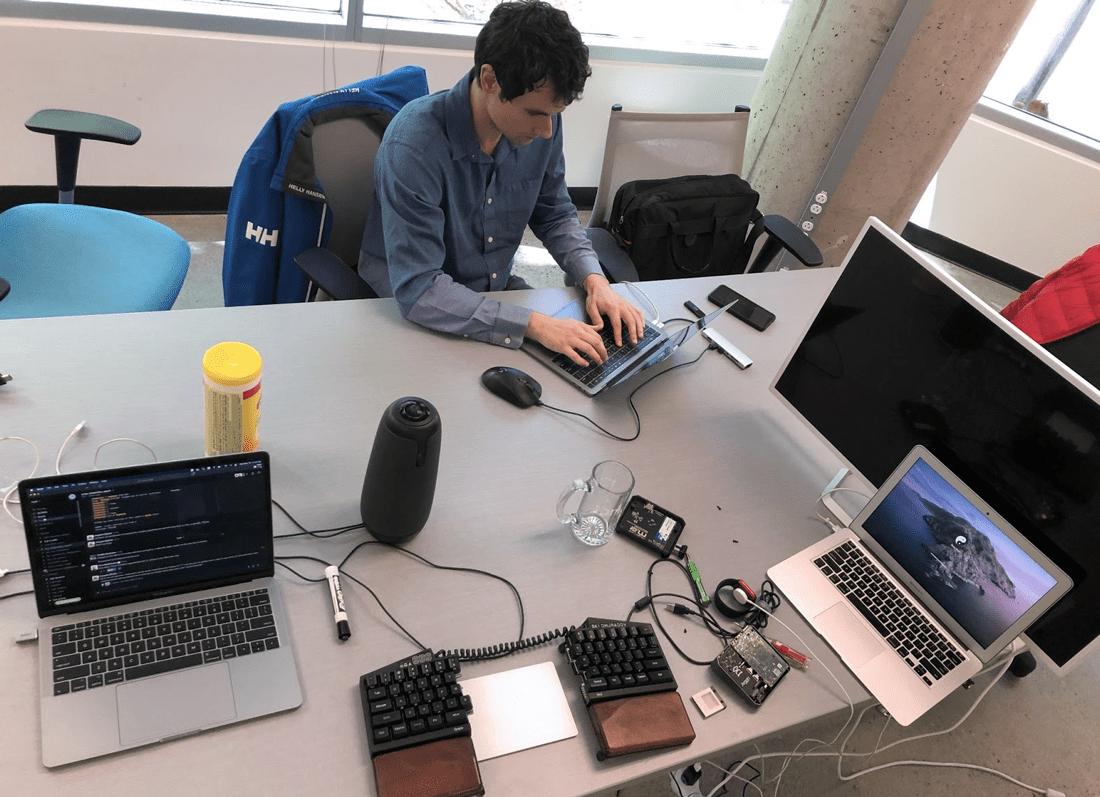
Figure 1: The set-up of the pAI project at the SIIM Hackathon.
The team took a picture of a plain X-ray film, which is still used in clinics in remote communities. Within two minutes the image was uploaded, sent to the Raspberry Pi, and results were returned.
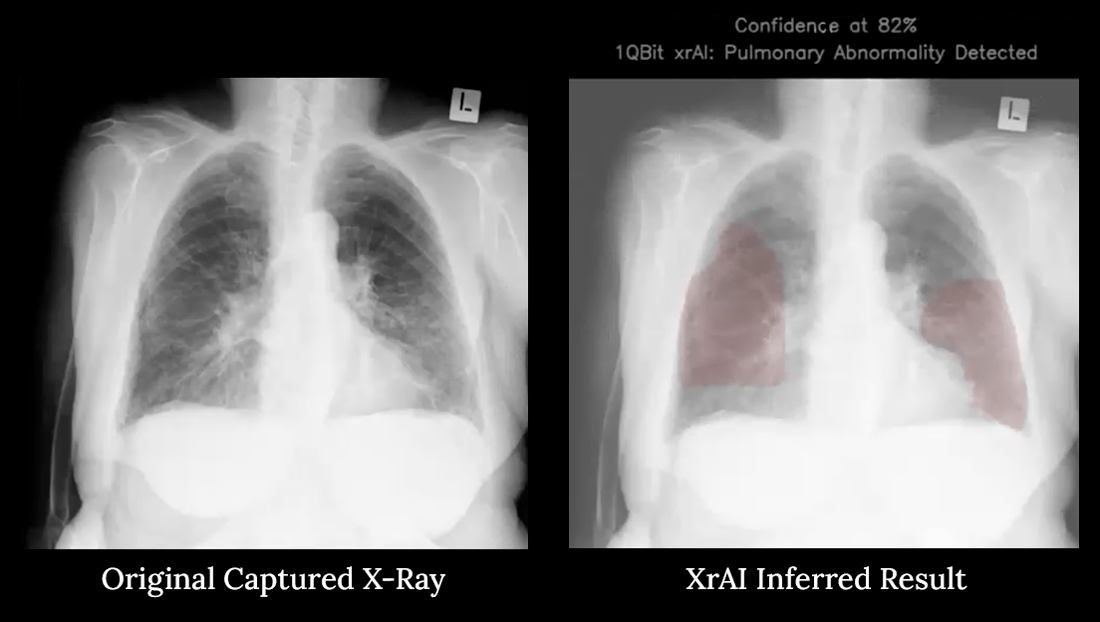
Figure 2: Demonstration of the pAI project. The heatmap in red indicates that the XrAI model was successfully implemented on a Raspberry Pi.
A heatmap is displayed, which helps the clinician to zone into the areas where an abnormality is detected. The deep learning model inferred that there was a high chance of a pulmonary abnormality present in this image. The interface on the smartphone appeared easy to use and the results were intuitive and informative.
Siong Ng, Healthcare Product Director at 1QBit, states that “This work opens up the possibility of deploying imaging-based AI to enable patient care in areas of the world with limited internet infrastructure. Our team will be working to improve the AI capability to indicate the type of pulmonary and pleural abnormality as well as optimizing the run-time components to deliver the inference faster.”
Siong continues, “Imagine the impact this would have for patient care, where a clinician working in a remote community can expedite the treatment decision with the help of this AI.”
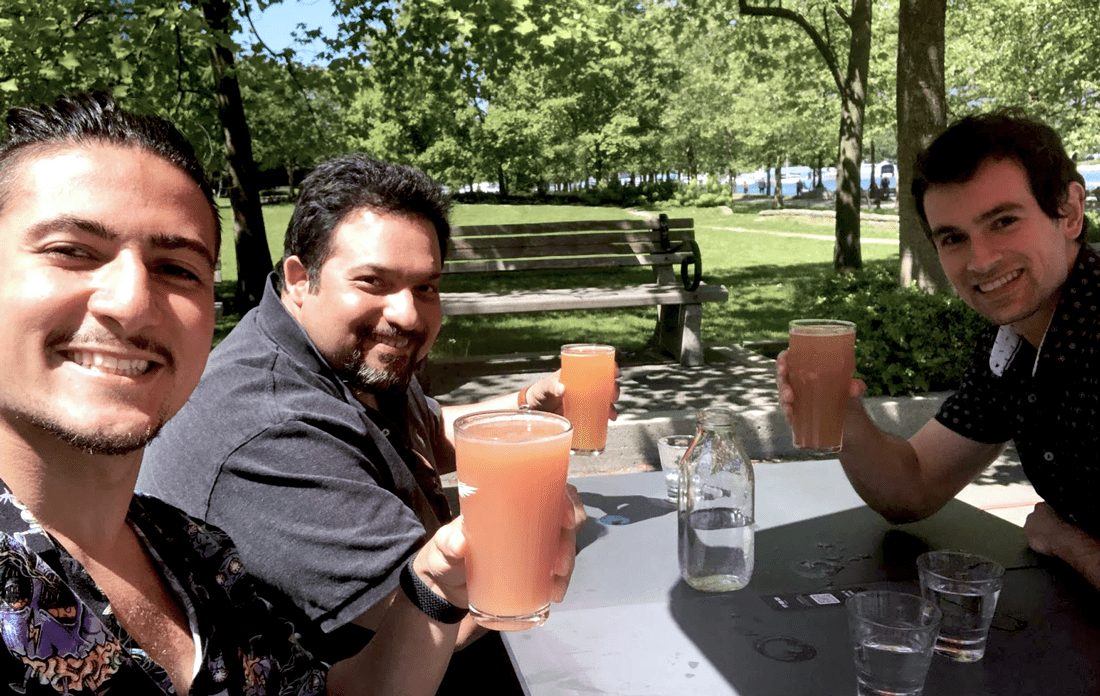
Figure 3: The 1QBit team celebrating their second-place finish at the Hackathon.
The team will continue to improve the functionality of pAI by optimizing the algorithm. They believe they can improve the time-to-results down from two minutes to about 30 seconds or less.
For more information on the pAI project or XrAI, please reach out to us at healthcare@1qbit.com.
Subscribe to the 1QBit Blog to keep up with other articles in health care and other topics related to machine learning and quantum computing applications.
References
1 A. P. Brady, “Error and discrepancy in radiology: inevitable or avoidable?” Insights Imaging, 8(1): 171–182, (2017).2 World Health Organization, “Communicating Radiation Risks in Pediatric Imaging,” Chapt. 1: 16, (2016).