Simon Verret is a scientist at 1QBit, solving some of the most challenging problems in machine learning. He first heard about 1QBit during an event organized by the Institut Quantique, Université de Sherbrooke, where he completed his Ph.D.
At the event, 1QBit CEO & Co-Founder Andrew Fursman discussed 1QBit’s vision of why Sherbrooke was chosen as a research hub. Simon has a shared interest in advanced computing, both classical and quantum, and knew that he wanted to work for 1QBit.
"1QBit is involved in the latest advances in both quantum physics and deep learning which are my two fields of expertise. Outside of academia, it is typically very difficult to be able to work on fundamental aspects of just one of these advanced topics, let alone both."
Almost a year later while working on his postdoc, Simon met Dominic Marchand, Head of Research and Partnerships at 1QBit. Dominic and Simon connected right away and they have been collaborating on research together at 1QBit ever since.
We sat down with Simon to explore what he is currently working on, why his research is important, and what he believes is the future of quantum computing.
What are you currently working on?
I work on machine learning algorithms called generative models which are used to synthesize fake data from a distribution. The idea is that forcing the model to generate fake data will result in it discovering the underlying real key features of the distribution automatically. Using generative models has many applications in machine learning because it enables more robust and reliable algorithms.
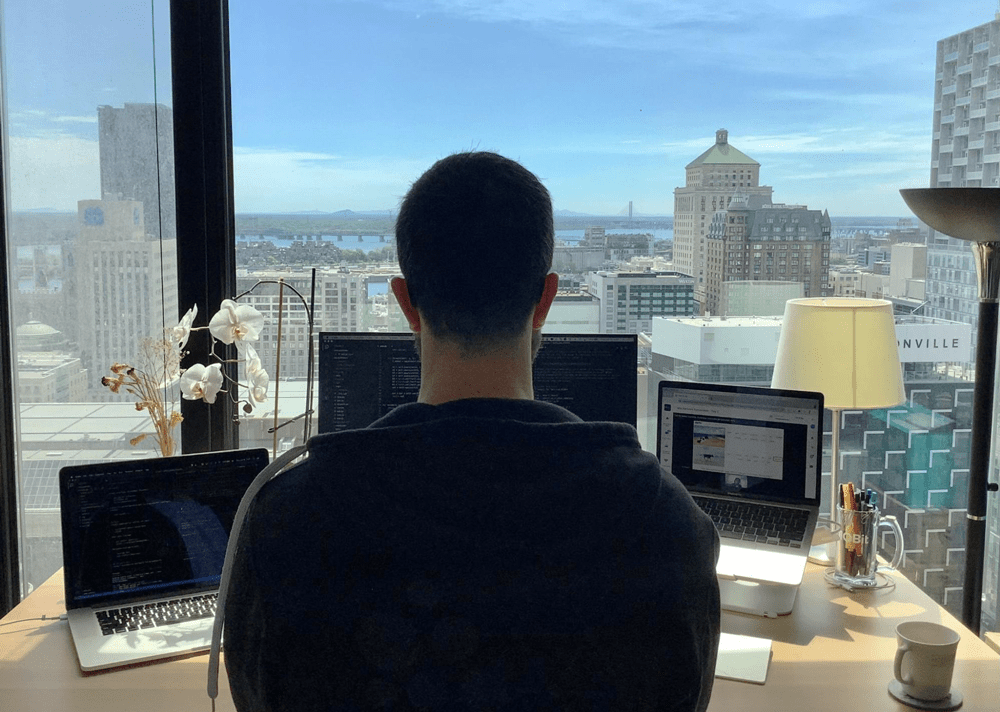
Simon Verret working from home in Montreal, Quebec.
What excites you about your work?
1QBit is involved in the latest advances in both quantum physics and deep learning which are my two fields of expertise. Outside of academia, it is typically very difficult to be able to work on fundamental aspects of just one of these advanced topics, let alone both. Being surrounded by experts in these fields and being encouraged to understand cutting-edge new concepts is a real privilege.
Do you have an analogy to help us understand your work?
Lately, we have been interested in reversing diffusion processes. When you put a drop of food colouring in water, it evolves through very complicated and beautiful structures before mixing completely and becoming homogeneously coloured water. The mathematical description of the diffusion process is well understood and widely used to model physical systems. The twist is that you can work these equations in reverse: Start from homogeneously coloured water and rewind time to get beautiful structures. In other words, start from noise, and get structure. This is generative modelling. By tuning the equations properly, it was recently discovered that one can generate fully realistic pictures of people, cars, churches, bedrooms, and more with this method. We are now exploring other applications of these principles, and how to make them more efficient.
What question or challenge were you setting out to address when you started this work?
This is what I find great about 1QBit’s approach. Our initial goal is pure exploration; the challenges and questions are left open. We make sure to understand the questions that have been answered so far, and the details of how to apply the latest methods. Then we look to apply these methods to otherwise intractable problems.
Why is your research important and what are the possible real-world applications?
The broader field of generative modelling already has many promising applications in the entertainment business. It can assist content creators by generating the highly detailed parts of their vision; for example, explosions, smoke, water, body movements, or even simplistic musical themes. This is very useful for movie and video game creation. While such use cases multiply, it is still not clear which of these algorithms are the most flexible and efficient. Outside of the entertainment industry, these new algorithms have already improved the robustness and reliability of older machine learning algorithms. This is necessary to reach reliable artificial intelligence (AI) for more sensitive applications like driverless cars, financial previsions, medical diagnostics, and many other applications.
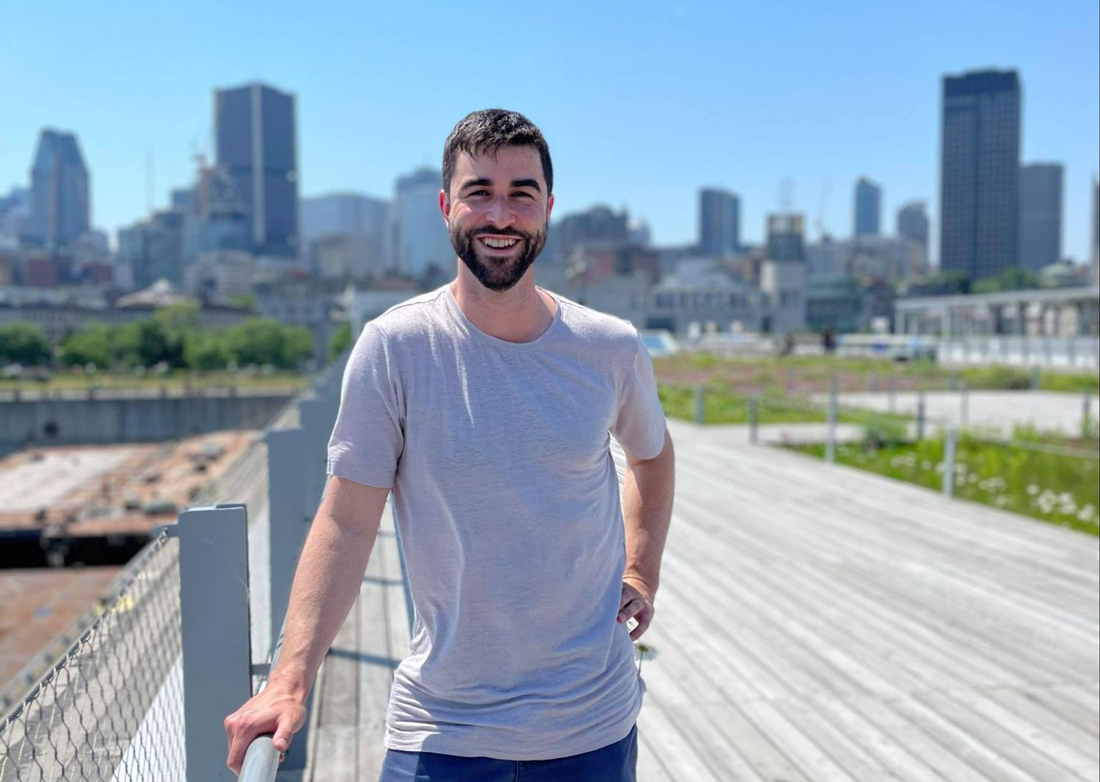
Simon exploring Quebec.
What kind of response has your research received?
So far, we are simply catching up with the very fast-paced research from the machine learning community. This project is one of the more far-sighted projects at 1QBit, and we expect things to get really interesting in the coming months.
What are the next steps for this work?
By understanding the algorithms better, we will be able to identify key limitations. We then want to improve them directly or better, cast them in a form where hardware acceleration or quantum computing can lead to a computational advantage. This last idea is particularly promising, because some of the algorithms we consider are inspired from microscopic physical processes, and quantum computers might grant a remarkable advantage.
What is the future of quantum computing?
Quantum computing is evolving on many fronts all at the same time. It is not like for classical algorithms, where you know what languages are well established, what libraries have been tested, which hardware architecture is compatible, and what performance can be expected. These aspects all have to be explored and refined together for quantum computing. I would say that quantum computing is likely to progress slowly for some undetermined time and then will rapidly burst into multiple applications when all the foundational tools are established.